Data is the lifeblood of every successful organization. It shares a unique view into the way your business operates and helps guide you toward a more efficient and prosperous future. With so much emphasis placed on the collection and analysis of data, there is precious little done with your unstructured data.
The Two Sides of Data
When it comes to data, many people fail to see the bigger picture. They know data is important, but they lack an understanding of how it’s used and where it comes from. As long as the data can tell a coherent story, leadership is content.
However, organizations are largely sharing a Cliff Notes version of their operations because of their singular focus on structured data. For businesses to truly improve and reach their potential, they need to understand both sides of data.
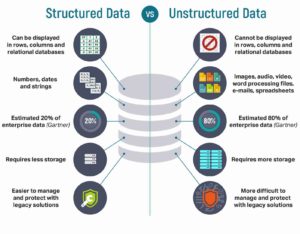
Source: Lawtomated
Structured Data
From a practical standpoint, the focus on structured data makes sense. Structured data is formatted in a uniform manner (e.g., categorized by predefined fields), making it easy for data scientists to search through, sort, and analyze against one another.
There is no question as to the context, meaning no nuance or variability to the results. This makes for straightforward analysis that can be performed relatively quickly — the core tenet of machine learning initiatives.
So what’s wrong with companies focusing their ML initiatives on structured data? It’s that organizations are only leveraging structured data — a fraction of the data available to them. You can still deliver results this way, but it will never provide a complete picture of your data.
Unstructured Data
So why is unstructured data getting the cold shoulder? The simple answer is convenience. Unstructured data does not come from an easy-to-search database, but rather from things like emails, phone calls, presentations, videos and much more.
When it comes to mining these sources for usable data, it’s not quite so quick and easy. Sure, you can search documents for specific text, but what does that really tell you? Beyond word or phrase frequency, not much else.
Unstructured data lacks context. So for you to glean any value from it, you must place it within the proper context. Humans can do it, but reading and analyzing text is a tedious and time-consuming task. Where do you go from here?
Integrating Natural Language Processing into Your AI
Language is extremely complex, but it creates depth and understanding of information that we cannot gather anywhere else. Thus, it would be foolish to ignore the sheer volume of unstructured data hidden in plain sight — especially when it contains 80% of your data load.
Natural language processing (NLP) enables you to focus on this unstructured data and address inefficiencies that have long held your organization back. However, like with any AI initiative, results are not a given. Success with NLP means careful integration into your larger AI plans with a clearly defined purpose and end goal.
NL Relies on Strategic Application
Whether you rely upon structured or unstructured data for your analysis, the best applications of AI are rooted in clear strategy. Any lack of strategy will render your AI useless, and is a big reason why 85% of AI initiatives don’t even get off the ground.
When it comes to natural language understanding, there are countless ways to leverage it. Every situation is unique in its own way, but there are several tried and true NL strategies that you can emulate.
You are only scratching the surface of your data and AI potential. Capture both sides of data, and there is no limit to how efficient you can become.